Second year
In this page you will find the description of the different second-year trainings offered within the Master ACSYON.
The three trainings of the second year of the Master Acsyon
Click on the boxes above to discover the corresponding training :
Local M2
This training is dedicated to english-speaker students willing to pursue their academic training at the University of Limoges. All courses are given in english and at the University of Limoges.
EUR M2
This training is dedicated to english-speaker students. It is a variant of the local training, with a special option related to the TACTIC Graduate School. It is the natural follow-up of the EUR M1 training. All courses are given in english at the University of Limoges.
MIX M2
This training is dedicated to french- and english-speaker students. It is the follow-up of the MIX M1 training. This training is addressed to students of the mechatronic training of ENSIL-ENSCI in Limoges. Three courses are delivered in english at the University of Limoges ; the rest of the training is ensured by ENSIL-ENSCI in Limoges, within the mechatronic training.
List of courses offered by M2 ACSYON
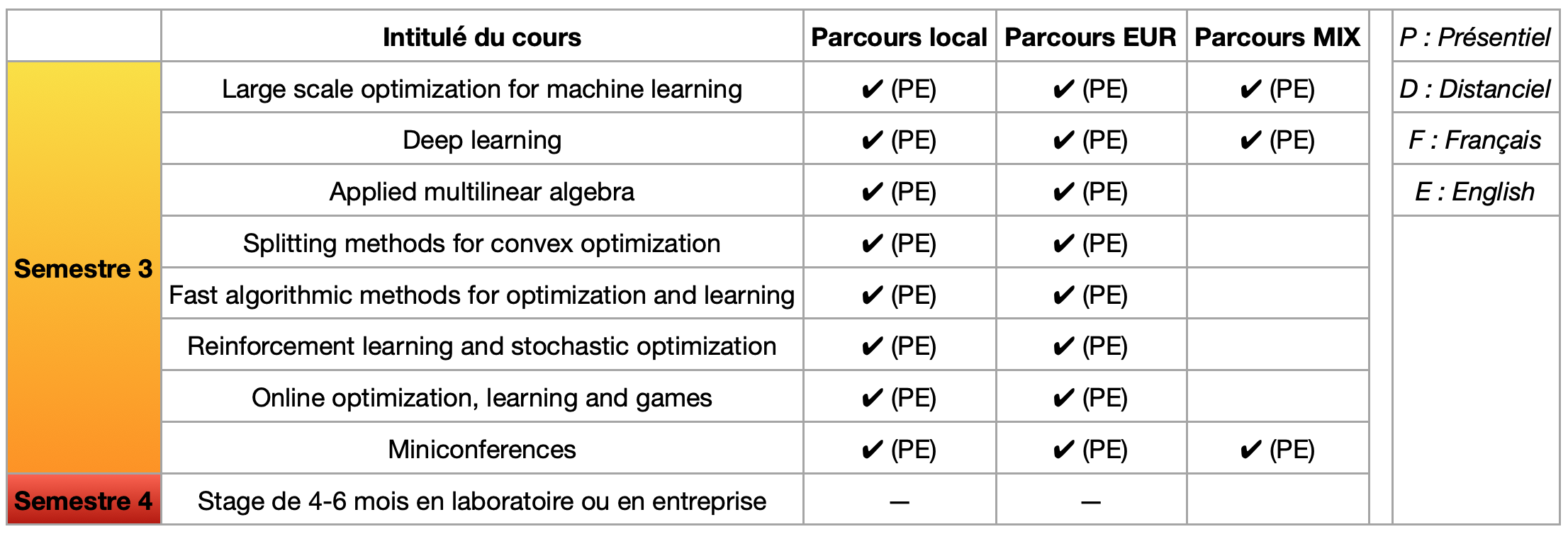
Description of the courses of the third semester
Optimization for large-scale machine learning
Prérequis
Optimization basics, linear algebra, convex geometry
Mots clés
Large-scale nonlinear optimization, big data optimization
Deep learning
Prérequis
Basics on numerical linear algebra, differential calculus and machine learning basics, Python
Mots clés
Neural network, algorithm, supervised learning, unsupervised learning, gradient descent
Applied multilinear algebra
Prérequis
Linear algebra, numerical matrix analysis, normed vector space, bilinear algebra, Euclidean spaces, differential calculus in R^n
Mots clés
Tensors, rank of tensors, singular value decomposition (HOSVD), tensor compression algorithm
Splitting methods for convex optimization
Several practical works (on Matlab or Pyhton) are performed during the semester in order to solve some standard problems that can be found in machine learning and data science, such as LASSO, consensus optimization, matrix decomposition problem, risk-averse optimization, clustering, image restoring (denoising, deblurring, inpainting), etc.
Prérequis
Convex analysis, topology, differential calculus, linear algebra and optimization basics
Mots clés
Maximal monotone set-values maps and resolvents, firmly non expansive and averaged operators, fixed-point algorithm, Krasnoselskii-Mann algorithm, projection operator, proximal operator, proximal point algorithm, proximal gradient algorithm (forward-backward), Douglas-Rachford algorithm, Davis-Yin algorithm, Lagrangian duality, primal-dual algorithms, method of multipliers, ADMM
Fast algorithmic methods for optimization and learning
The second part of this course will be devoted to the link between continuous differential equations and algorithms in optimization (obtained by explicit/implicit temporal discretization). This back and forth between continuous and discrete dynamics allowed us to propose efficient and fast algorithms in optimization. This include:
– damped inertial gradient method for understanding and extending Nesterov accelerated gradient method
– Hessian-driven damping for speeding up and damping oscillations of optimization algorithms,
– Fast algorithms in convex optimization based on inertial gradient-based dynamics
– Asymptotic vanishing damping and its link with Nesterov acceleration.
Applications will be given in image processing such as image denoising, image deconvolution, image inpainting, motion estimation and image segmentation.
Prérequis
Linear algebra, topology and differential calculus in R^n, convex analysis, optimization basics
Mots clés
First order optimization algorithms, ISTA, FISTA, Nesterov acceleration, damped inertial gradient methods, Hessian-driven damping algorithms, asymptotic vanishing damping
Reinforcement learning and stochastic optimization
Prérequis
Optimization basics, practical optimization and stochastic processes
Online optimization, learning and games
Mots clés
Game theory, learning, optimization, gradient-based techniques
Miniconferences
Mots clés
Thematic conferences, practical cases, applied sciences, business